Data-driven knowledge acquisition, validation, and transformation into HL7 Arden Syntax
Journal article
Authors | Hussain, Maqbool, Afzal, Muhammad, Ali, Taqdir, Ali, Rahman, Khan, Wajahat Ali, Jamshed, Arif, Lee, Sungyoung, Kang, Byeong Ho and Latif, Khalid |
---|---|
Abstract | The objective of this study is to help a team of physicians and knowledge engineers acquire clinical knowledge from existing practices datasets for treatment of head and neck cancer, to validate the knowledge against published guidelines, to create refined rules, and to incorporate these rules into clinical workflow for clinical decision support. A team of physicians (clinical domain experts) and knowledge engineers adapt an approach for modeling existing treatment practices into final executable clinical models. For initial work, the oral cavity is selected as the candidate target area for the creation of rules covering a treatment plan for cancer. The final executable model is presented in HL7 Arden Syntax, which helps the clinical knowledge be shared among organizations. We use a data-driven knowledge acquisition approach based on analysis of real patient datasets to generate a predictive model (PM). The PM is converted into a refined-clinical knowledge model (R-CKM), which follows a rigorous validation process. The validation process uses a clinical knowledge model (CKM), which provides the basis for defining underlying validation criteria. The R-CKM is converted into a set of medical logic modules (MLMs) and is evaluated using real patient data from a hospital information system. We selected the oral cavity as the intended site for derivation of all related clinical rules for possible associated treatment plans. A team of physicians analyzed the National Comprehensive Cancer Network (NCCN) guidelines for the oral cavity and created a common CKM. Among the decision tree algorithms, chi-squared automatic interaction detection (CHAID) was applied to a refined dataset of 1229 patients to generate the PM. The PM was tested on a disjoint dataset of 739 patients, which gives 59.0% accuracy. Using a rigorous validation process, the R-CKM was created from the PM as the final model, after conforming to the CKM. The R-CKM was converted into four candidate MLMs, and was used to evaluate real data from 739 patients, yielding efficient performance with 53.0% accuracy. Data-driven knowledge acquisition and validation against published guidelines were used to help a team of physicians and knowledge engineers create executable clinical knowledge. The advantages of the R-CKM are twofold: it reflects real practices and conforms to standard guidelines, while providing optimal accuracy comparable to that of a PM. The proposed approach yields better insight into the steps of knowledge acquisition and enhances collaboration efforts of the team of physicians and knowledge engineers. |
Keywords | Knowledge acquisition, Knowledge validation, Prediction models, Clinical guidelines, Clinical decision support systems, HL7 Arden Syntax |
Year | 2015 |
Journal | Artificial Intelligence in Medicine |
Journal citation | 92, pp. 51-70 |
Publisher | Elsevier BV |
ISSN | 0933-3657 |
Digital Object Identifier (DOI) | https://doi.org/10.1016/j.artmed.2015.09.008 |
Web address (URL) | http://hdl.handle.net/10545/624843 |
https://www.elsevier.com/tdm/userlicense/1.0/ | |
hdl:10545/624843 | |
Publication dates | 28 Oct 2015 |
Publication process dates | |
Deposited | 29 May 2020, 12:17 |
Accepted | 15 Sep 2015 |
Rights | © 2015 Elsevier B.V. All rights reserved. |
Contributors | Kyung Hee University, Seocheon-dong, Giheung-gu, Yongin-si 446-701, Gyeonggi-do, Republic of Korea, Shaukat Khanum Memorial Cancer Hospital and Research Centre, 7A Block R-3, M.A. Johar Town, Lahore 54782, Pakistan, University of Tasmania, Hobart 7001, Tasmania, Australia and COMSATS Institute of Information Technology, Park Road, Islamabad 45550, Pakistan |
File | File Access Level Open |
https://repository.derby.ac.uk/item/92y5q/data-driven-knowledge-acquisition-validation-and-transformation-into-hl7-arden-syntax
Download files
65
total views0
total downloads3
views this month0
downloads this month
Export as
Related outputs
Transformer-based active learning for multi-class text annotation and classification
Hussain, M. 2024. Transformer-based active learning for multi-class text annotation and classification. Digital Health. pp. 1-21. https://doi.org/10.1177/20552076241287357Optimizing Aerospace Product Maintenance A Novel Multi-Modal Knowledge Graph and LLM Approach for Enhanced Decision Support
Awill, R., Khan, W., Hussain, M. and Anderson, B. 2024. Optimizing Aerospace Product Maintenance A Novel Multi-Modal Knowledge Graph and LLM Approach for Enhanced Decision Support. The Extended Semantic Web Conference 2024: Fabrics of Knowledge: Knowledge Graphs and Generative AI. The Extended Semantic Web .STK3 higher expression association with clinical characteristics in intrinsic subtypes of breast cancer invasive ductal carcinoma patients
Rukhsana, R., Supty, A.T., Hussain, M. and Lee, Y. 2024. STK3 higher expression association with clinical characteristics in intrinsic subtypes of breast cancer invasive ductal carcinoma patients. Breast Cancer Research and Treatment. 206, p. 119–129. https://doi.org/10.1007/s10549-024-07248-3A unified graph model based on molecular data binning for disease subtyping
Hassan Zada, M., Yuan, B, Khan, W., Anjum, A., Reiff-Marganiec, S. and Saleem, R. 2022. A unified graph model based on molecular data binning for disease subtyping. Journal of Biomedical Informatics. pp. 1-24. https://doi.org/10.1016/j.jbi.2022.104187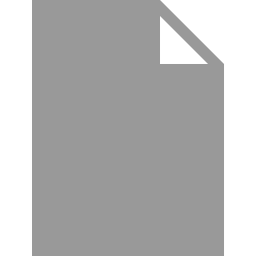
Large-scale Data Integration Using Graph Probabilistic Dependencies (GPDs)
Zada, Muhammad Sadiq Hassan, Yuan, Bo, Anjum, Ashiq, Azad, Muhammad Ajmal, Khan, Wajahat Ali and Reiff-Marganiec, Stephan 2020. Large-scale Data Integration Using Graph Probabilistic Dependencies (GPDs). IEEE. https://doi.org/10.1109/bdcat50828.2020.00028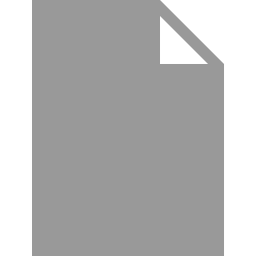
Tweets classification and sentiment analysis for personalized tweets recommendation
Batool, Rabia, Satti, Fahad Ahmed, Hussain, Jamil, Khan, Wajahat Ali, Khan, Adil Mehmood and Hayat, Bashir 2020. Tweets classification and sentiment analysis for personalized tweets recommendation. Complexity in Deep Neural Networks. 2020. https://doi.org/10.1155/2020/8892552Entropy Based Features Distribution for Anti-DDoS Model in SDN
Raja Majid Ali Ujjan, Zeeshan Pervez, Keshav Dahal, Wajahat Ali Khan, Asad Masood Khattak and Bashir Hayat 2021. Entropy Based Features Distribution for Anti-DDoS Model in SDN. Sustainability. 13 (3), pp. 1-27. https://doi.org/10.3390/su13031522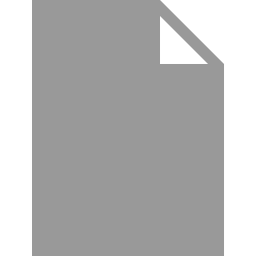
Ubiquitous health profile (UHPr): a big data curation platform for supporting health data interoperability
Satti, Fahad Ahmed, Ali, Taqdir, Hussain, Jamil, Khan, Wajahat Ali, Khattak, Asad Masood and Lee, Sungyoung 2020. Ubiquitous health profile (UHPr): a big data curation platform for supporting health data interoperability. Computing. https://doi.org/10.1007/s00607-020-00837-2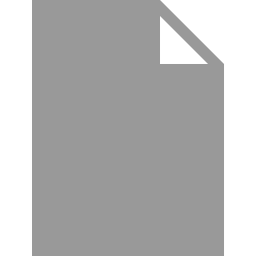
The mining minds digital health and wellness framework
Banos, Oresti, Bilal Amin, Muhammad, Khan, Wajahat Ali, Afzal, Muhammad, Hussain, Maqbool, Kang, Byeong Ho and Lee, Sungyong 2016. The mining minds digital health and wellness framework. BioMedical Engineering OnLine. 15 (S1). https://doi.org/10.1186/s12938-016-0179-9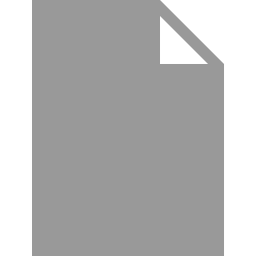
Multi-model-based interactive authoring environment for creating shareable medical knowledge
Ali, Taqdir, Hussain, Maqbool, Khan, Wajahat Ali, Afzal, Muhammad, Hussain, Jamil, Ali, Rahman, Hassan, Waseem, Jamshed, Arif, Kang, Byeong Ho and Lee, Sungyoung 2017. Multi-model-based interactive authoring environment for creating shareable medical knowledge. Computer Methods and Programs in Biomedicine. 150, pp. 41-72. https://doi.org/10.1016/j.cmpb.2017.07.010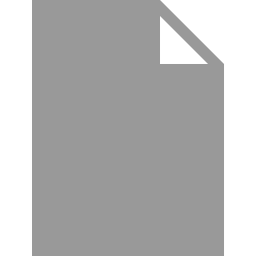
An adaptive semantic based mediation system for data interoperability among health information systems
Khan, Wajahat Ali, Khattak, Asad Masood, Hussain, Maqbool, Amin, Muhammad Bilal, Afzal, Muhammad, Nugent, Christopher and Lee, Sungyoung 2014. An adaptive semantic based mediation system for data interoperability among health information systems. Journal of Medical Systems. 38 (8). https://doi.org/10.1007/s10916-014-0028-y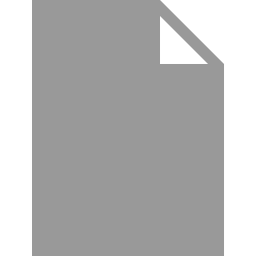
Mapping evolution of dynamic web ontologies
Khattak, A.M., Pervez, Z., Khan, Wajahat Ali, Khan, A.M., Latif, K. and Lee, S.Y. 2015. Mapping evolution of dynamic web ontologies. Information Sciences. 303, pp. 101-119. https://doi.org/10.1016/j.ins.2014.12.040The intelligent medical platform: a novel dialogue-based platform for health-care services
Taqdir Ali, Jamil Hussain, Muhammad Bilal Amin, Musarrat Hussain, Usman Akhtar, Wajahat Ali Khan, Sungyoung Lee, Byeong Ho Kang, Maqbool Hussain, Muhammad Afzal, Hyeong Won Yu, Ubaid Ur Rehman, Ho-Seong Han, June Young Choi and Arif Jamshed The intelligent medical platform: a novel dialogue-based platform for health-care services. Computer. https://doi.org/10.1109/mc.2019.2924393Exploring the dominant features of social media for depression detection
Hussain, J., Satti, F.A., Afzal, M., Khan, W.A., Bilal, H.S.M., Ansaar, M.Z., Ahmad, H.F., Hur, T., Bang, J., Kim, J.-I., Park, G.H., Seung, H., Lee, S. and Khan, W. Exploring the dominant features of social media for depression detection. Journal of Information Science. https://doi.org/10.1177/0165551519860469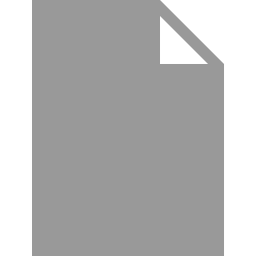