Optimizing Aerospace Product Maintenance A Novel Multi-Modal Knowledge Graph and LLM Approach for Enhanced Decision Support
Conference paper
Authors | Awill, R., Khan, W., Hussain, M. and Anderson, B. |
---|---|
Type | Conference paper |
Abstract | Siloed and inaccessible repair knowledge hinders the efficient maintenance of critical Turbine Engine components in the aerospace industry. This research introduces a novel multi-modal knowledge graph, leveraging Natural Language Processing (NLP) and Large Language Models (LLMs) to extract and structure repair rules from unstructured documents into a 131-node, 148-relationship graph. This advancement enables immediate access to essential information and facilitates datadriven decision-making, enhancing repair accuracy and efficiency. Implemented at AddQual Ltd., the knowledge graph reduced information retrieval times by 70%, increased repair speed by 20%, and is projected to yield 20% annual cost savings. These results highlight the transformative potential of integrating AI with knowledge graphs in aerospace maintenance. Future work will focus on advancing robust data validation frameworks and developing adaptive AI algorithms, extending the benefits across the aerospace sector and beyond. |
Keywords | Knowledge Graph; Multi-Modal; Turbine Repair; Decision Support; Aerospace; Text2Cypher |
Year | 2024 |
Conference | The Extended Semantic Web Conference 2024: Fabrics of Knowledge: Knowledge Graphs and Generative AI |
Publisher | The Extended Semantic Web |
Web address (URL) | https://2024.eswc-conferences.org/wp-content/uploads/2024/05/77770127.pdf |
Output status | Published |
Publication dates | 29 May 2024 |
Publication process dates | |
Deposited | 27 Jun 2024 |
https://repository.derby.ac.uk/item/q70z5/optimizing-aerospace-product-maintenance-a-novel-multi-modal-knowledge-graph-and-llm-approach-for-enhanced-decision-support
136
total views0
total downloads14
views this month0
downloads this month
Export as
Related outputs
Transformer-based active learning for multi-class text annotation and classification
Hussain, M. 2024. Transformer-based active learning for multi-class text annotation and classification. Digital Health. pp. 1-21. https://doi.org/10.1177/20552076241287357A unified graph model based on molecular data binning for disease subtyping
Hassan Zada, M., Yuan, B, Khan, W., Anjum, A., Reiff-Marganiec, S. and Saleem, R. 2022. A unified graph model based on molecular data binning for disease subtyping. Journal of Biomedical Informatics. pp. 1-24. https://doi.org/10.1016/j.jbi.2022.104187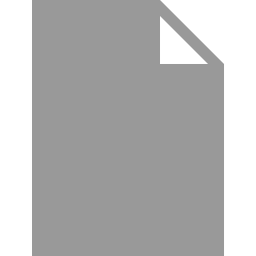
Large-scale Data Integration Using Graph Probabilistic Dependencies (GPDs)
Zada, Muhammad Sadiq Hassan, Yuan, Bo, Anjum, Ashiq, Azad, Muhammad Ajmal, Khan, Wajahat Ali and Reiff-Marganiec, Stephan 2020. Large-scale Data Integration Using Graph Probabilistic Dependencies (GPDs). IEEE. https://doi.org/10.1109/bdcat50828.2020.00028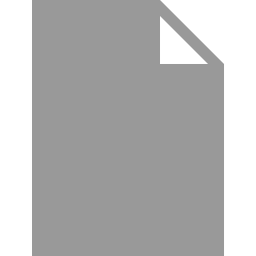
Tweets classification and sentiment analysis for personalized tweets recommendation
Batool, Rabia, Satti, Fahad Ahmed, Hussain, Jamil, Khan, Wajahat Ali, Khan, Adil Mehmood and Hayat, Bashir 2020. Tweets classification and sentiment analysis for personalized tweets recommendation. Complexity in Deep Neural Networks. 2020. https://doi.org/10.1155/2020/8892552Entropy Based Features Distribution for Anti-DDoS Model in SDN
Raja Majid Ali Ujjan, Zeeshan Pervez, Keshav Dahal, Wajahat Ali Khan, Asad Masood Khattak and Bashir Hayat 2021. Entropy Based Features Distribution for Anti-DDoS Model in SDN. Sustainability. 13 (3), pp. 1-27. https://doi.org/10.3390/su13031522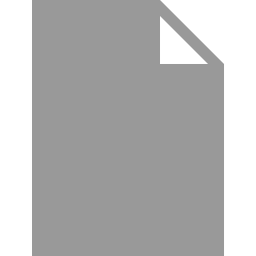
Ubiquitous health profile (UHPr): a big data curation platform for supporting health data interoperability
Satti, Fahad Ahmed, Ali, Taqdir, Hussain, Jamil, Khan, Wajahat Ali, Khattak, Asad Masood and Lee, Sungyoung 2020. Ubiquitous health profile (UHPr): a big data curation platform for supporting health data interoperability. Computing. https://doi.org/10.1007/s00607-020-00837-2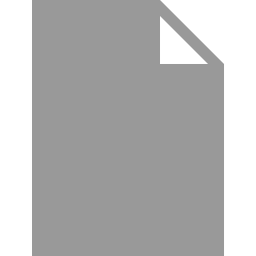
Data-driven knowledge acquisition, validation, and transformation into HL7 Arden Syntax
Hussain, Maqbool, Afzal, Muhammad, Ali, Taqdir, Ali, Rahman, Khan, Wajahat Ali, Jamshed, Arif, Lee, Sungyoung, Kang, Byeong Ho and Latif, Khalid 2015. Data-driven knowledge acquisition, validation, and transformation into HL7 Arden Syntax. Artificial Intelligence in Medicine. 92, pp. 51-70. https://doi.org/10.1016/j.artmed.2015.09.008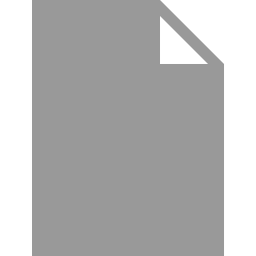
The mining minds digital health and wellness framework
Banos, Oresti, Bilal Amin, Muhammad, Khan, Wajahat Ali, Afzal, Muhammad, Hussain, Maqbool, Kang, Byeong Ho and Lee, Sungyong 2016. The mining minds digital health and wellness framework. BioMedical Engineering OnLine. 15 (S1). https://doi.org/10.1186/s12938-016-0179-9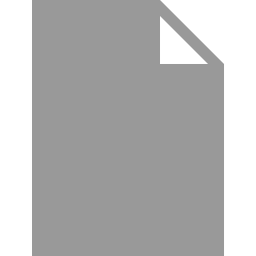
Multi-model-based interactive authoring environment for creating shareable medical knowledge
Ali, Taqdir, Hussain, Maqbool, Khan, Wajahat Ali, Afzal, Muhammad, Hussain, Jamil, Ali, Rahman, Hassan, Waseem, Jamshed, Arif, Kang, Byeong Ho and Lee, Sungyoung 2017. Multi-model-based interactive authoring environment for creating shareable medical knowledge. Computer Methods and Programs in Biomedicine. 150, pp. 41-72. https://doi.org/10.1016/j.cmpb.2017.07.010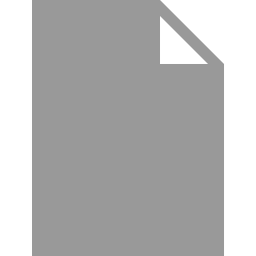
An adaptive semantic based mediation system for data interoperability among health information systems
Khan, Wajahat Ali, Khattak, Asad Masood, Hussain, Maqbool, Amin, Muhammad Bilal, Afzal, Muhammad, Nugent, Christopher and Lee, Sungyoung 2014. An adaptive semantic based mediation system for data interoperability among health information systems. Journal of Medical Systems. 38 (8). https://doi.org/10.1007/s10916-014-0028-y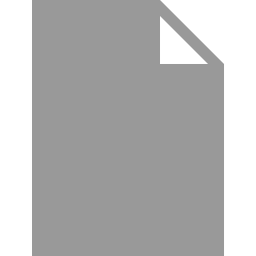
Mapping evolution of dynamic web ontologies
Khattak, A.M., Pervez, Z., Khan, Wajahat Ali, Khan, A.M., Latif, K. and Lee, S.Y. 2015. Mapping evolution of dynamic web ontologies. Information Sciences. 303, pp. 101-119. https://doi.org/10.1016/j.ins.2014.12.040The intelligent medical platform: a novel dialogue-based platform for health-care services
Taqdir Ali, Jamil Hussain, Muhammad Bilal Amin, Musarrat Hussain, Usman Akhtar, Wajahat Ali Khan, Sungyoung Lee, Byeong Ho Kang, Maqbool Hussain, Muhammad Afzal, Hyeong Won Yu, Ubaid Ur Rehman, Ho-Seong Han, June Young Choi and Arif Jamshed The intelligent medical platform: a novel dialogue-based platform for health-care services. Computer. https://doi.org/10.1109/mc.2019.2924393Exploring the dominant features of social media for depression detection
Hussain, J., Satti, F.A., Afzal, M., Khan, W.A., Bilal, H.S.M., Ansaar, M.Z., Ahmad, H.F., Hur, T., Bang, J., Kim, J.-I., Park, G.H., Seung, H., Lee, S. and Khan, W. Exploring the dominant features of social media for depression detection. Journal of Information Science. https://doi.org/10.1177/0165551519860469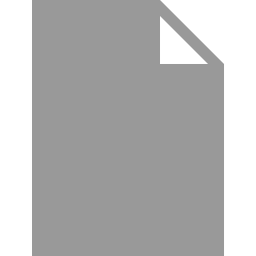