Acquiring guideline-enabled data driven clinical knowledge model using formally verified refined knowledge acquisition method
Journal article
Authors | Afzal, Muhammad, Malik, Khalid M., Ali, Taqdir, Ali Khan, Wajahat, Irfan, Muhammad, Jamshrf, Arif, Lee, Sungyoung and Hussain, Maqbool |
---|---|
Abstract | Background and Objective: Validation and verification are the critical requirements for the knowledge acquisition method of the clinical decision support system (CDSS). After acquiring the medical knowledge from diverse sources, the rigorous validation and formal verification process are required before creating the final knowledge model. Previously, we have proposed a hybrid knowledge acquisition method with the support of a rigorous validation process for acquiring medical knowledge from clinical practice guidelines (CPGs) and patient data for the treatment of oral cavity cancer. However, due to lack of formal verification process, it involves various inconsistencies in knowledge relevant to the formalism of knowledge, conformance to CPGs, quality of knowledge, and complexities of knowledge acquisition artifacts.Methods: This paper presents the refined knowledge acquisition (ReKA) method, which uses the Z formal verification process. The ReKA method adopts the verification method and explores the mechanism of theorem proving using the Z notation. It enhances a hybrid knowledge acquisition method to thwart the inconsistencies using formal verification.Results: ReKA adds a set of nine additional criteria to be used to have a final valid refined clinical knowledge model. These criteria ensure the validity of the final knowledge model concerning formalism of knowledge, conformance to GPGs, quality of the knowledge, usage of stringent conditions and treatment plans, and inconsistencies possibly resulting from the complexities. Evaluation, using four medical knowledge acquisition scenarios, shows that newly added knowledge in CDSS due to the additional criteria by the ReKA method always produces a valid knowledge model. The final knowledge model was also evaluated with 1229 oral cavity patient cases, which outperformed with an accuracy of 72.57% compared to a similar approach with an accuracy of 69.7%. Furthermore, the ReKA method identified a set of decision paths (about 47.8%) in the existing approach, which results in a final knowledge model with low quality, non-conformed from standard CPGs.Conclusion: ReKA refined the hybrid knowledge acquisition method by discovering the missing steps in the current validation process at the acquisition stage. As a formally proven method, it always yields a valid knowledge model having high quality, supporting local practices, and influenced by standard CPGs. Furthermore, the final knowledge model obtained from ReKA also preserves the performance such as the accuracy of the individual source knowledge models. |
Keywords | Knowledge acquisition; Clinical practice guidelines; Data driven knowledge acquisition; Cancer treatment plan; Clinical decision support system; Formal verification |
Year | 2020 |
Journal | Computer Methods and Programs in Biomedicine |
Publisher | Elsevier |
ISSN | 01692607 |
Digital Object Identifier (DOI) | https://doi.org/10.1016/j.cmpb.2020.105701 |
Web address (URL) | http://hdl.handle.net/10545/625145 |
hdl:10545/625145 | |
Publication dates | 19 Aug 2020 |
Publication process dates | |
Deposited | 01 Sep 2020, 12:02 |
Accepted | 05 Aug 2020 |
Rights | © 2020 Elsevier B.V. All rights reserved. |
Contributors | Sejong University, Seoul, South Korea, Oakland University, Rochester, MI, USA, Kyung Hee University, Republic of Korea, Shaukat Khanum Memorial Cancer Hospital and Research Centre, Lahore, Pakistan, National Guard-Health Affairs, King Abdulaziz Medical City Riyadh, Kingdom of Saudi Arabia and University of Derby |
File | File Access Level Open |
File |
https://repository.derby.ac.uk/item/94vz6/acquiring-guideline-enabled-data-driven-clinical-knowledge-model-using-formally-verified-refined-knowledge-acquisition-method
Download files
76
total views72
total downloads2
views this month1
downloads this month
Export as
Related outputs
Transformer-based active learning for multi-class text annotation and classification
Hussain, M. 2024. Transformer-based active learning for multi-class text annotation and classification. Digital Health. pp. 1-21. https://doi.org/10.1177/20552076241287357Optimizing Aerospace Product Maintenance A Novel Multi-Modal Knowledge Graph and LLM Approach for Enhanced Decision Support
Awill, R., Khan, W., Hussain, M. and Anderson, B. 2024. Optimizing Aerospace Product Maintenance A Novel Multi-Modal Knowledge Graph and LLM Approach for Enhanced Decision Support. The Extended Semantic Web Conference 2024: Fabrics of Knowledge: Knowledge Graphs and Generative AI. The Extended Semantic Web .STK3 higher expression association with clinical characteristics in intrinsic subtypes of breast cancer invasive ductal carcinoma patients
Rukhsana, R., Supty, A.T., Hussain, M. and Lee, Y. 2024. STK3 higher expression association with clinical characteristics in intrinsic subtypes of breast cancer invasive ductal carcinoma patients. Breast Cancer Research and Treatment. 206, p. 119–129. https://doi.org/10.1007/s10549-024-07248-3A unified graph model based on molecular data binning for disease subtyping
Hassan Zada, M., Yuan, B, Khan, W., Anjum, A., Reiff-Marganiec, S. and Saleem, R. 2022. A unified graph model based on molecular data binning for disease subtyping. Journal of Biomedical Informatics. pp. 1-24. https://doi.org/10.1016/j.jbi.2022.104187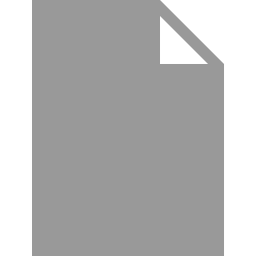
Large-scale Data Integration Using Graph Probabilistic Dependencies (GPDs)
Zada, Muhammad Sadiq Hassan, Yuan, Bo, Anjum, Ashiq, Azad, Muhammad Ajmal, Khan, Wajahat Ali and Reiff-Marganiec, Stephan 2020. Large-scale Data Integration Using Graph Probabilistic Dependencies (GPDs). IEEE. https://doi.org/10.1109/bdcat50828.2020.00028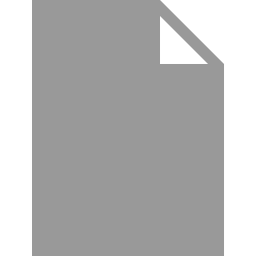
Tweets classification and sentiment analysis for personalized tweets recommendation
Batool, Rabia, Satti, Fahad Ahmed, Hussain, Jamil, Khan, Wajahat Ali, Khan, Adil Mehmood and Hayat, Bashir 2020. Tweets classification and sentiment analysis for personalized tweets recommendation. Complexity in Deep Neural Networks. 2020. https://doi.org/10.1155/2020/8892552Entropy Based Features Distribution for Anti-DDoS Model in SDN
Raja Majid Ali Ujjan, Zeeshan Pervez, Keshav Dahal, Wajahat Ali Khan, Asad Masood Khattak and Bashir Hayat 2021. Entropy Based Features Distribution for Anti-DDoS Model in SDN. Sustainability. 13 (3), pp. 1-27. https://doi.org/10.3390/su13031522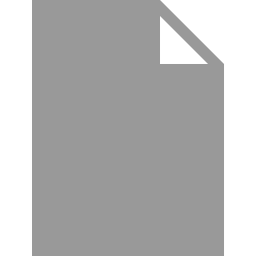
Ubiquitous health profile (UHPr): a big data curation platform for supporting health data interoperability
Satti, Fahad Ahmed, Ali, Taqdir, Hussain, Jamil, Khan, Wajahat Ali, Khattak, Asad Masood and Lee, Sungyoung 2020. Ubiquitous health profile (UHPr): a big data curation platform for supporting health data interoperability. Computing. https://doi.org/10.1007/s00607-020-00837-2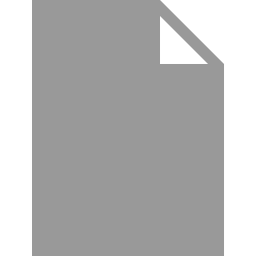
Data-driven knowledge acquisition, validation, and transformation into HL7 Arden Syntax
Hussain, Maqbool, Afzal, Muhammad, Ali, Taqdir, Ali, Rahman, Khan, Wajahat Ali, Jamshed, Arif, Lee, Sungyoung, Kang, Byeong Ho and Latif, Khalid 2015. Data-driven knowledge acquisition, validation, and transformation into HL7 Arden Syntax. Artificial Intelligence in Medicine. 92, pp. 51-70. https://doi.org/10.1016/j.artmed.2015.09.008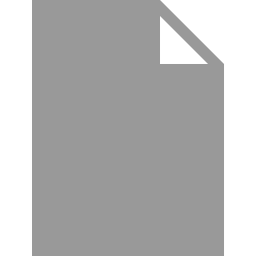
The mining minds digital health and wellness framework
Banos, Oresti, Bilal Amin, Muhammad, Khan, Wajahat Ali, Afzal, Muhammad, Hussain, Maqbool, Kang, Byeong Ho and Lee, Sungyong 2016. The mining minds digital health and wellness framework. BioMedical Engineering OnLine. 15 (S1). https://doi.org/10.1186/s12938-016-0179-9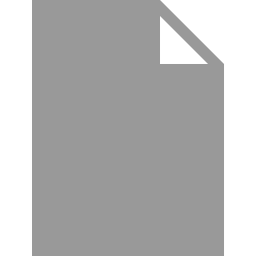
Multi-model-based interactive authoring environment for creating shareable medical knowledge
Ali, Taqdir, Hussain, Maqbool, Khan, Wajahat Ali, Afzal, Muhammad, Hussain, Jamil, Ali, Rahman, Hassan, Waseem, Jamshed, Arif, Kang, Byeong Ho and Lee, Sungyoung 2017. Multi-model-based interactive authoring environment for creating shareable medical knowledge. Computer Methods and Programs in Biomedicine. 150, pp. 41-72. https://doi.org/10.1016/j.cmpb.2017.07.010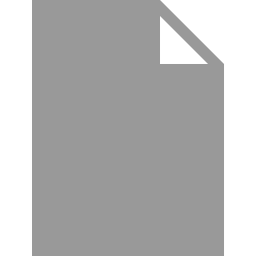
An adaptive semantic based mediation system for data interoperability among health information systems
Khan, Wajahat Ali, Khattak, Asad Masood, Hussain, Maqbool, Amin, Muhammad Bilal, Afzal, Muhammad, Nugent, Christopher and Lee, Sungyoung 2014. An adaptive semantic based mediation system for data interoperability among health information systems. Journal of Medical Systems. 38 (8). https://doi.org/10.1007/s10916-014-0028-y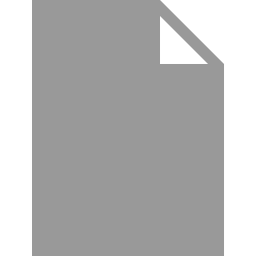