Data classification using the Dempster–Shafer method.
Journal article
Authors | Chen, Qi, Whitbrook, Amanda, Aickelin, Uwe and Roadknight, Chris |
---|---|
Abstract | In this paper, the Dempster–Shafer (D–S) method is used as the theoretical basis for creating data classification systems. Testing is carried out using three popular multiple attribute benchmark data-sets that have two, three and four classes. In each case, a subset of the available data is used for training to establish thresholds, limits or likelihoods of class membership for each attribute, and hence create mass functions that establish probability of class membership for each attribute of the test data. Classification of each data item is achieved by combination of these probabilities via Dempster’s rule of combination. Results for the first two data-sets show extremely high classification accuracy that is competitive with other popular methods. The third data-set is non-numerical and difficult to classify, but good results can be achieved provided the system and mass functions are designed carefully and the right attributes are chosen for combination. In all cases, the D–S method provides comparable performance to other more popular algorithms, but the overhead of generating accurate mass functions increases the complexity with the addition of new attributes. Overall, the results suggest that the D–S approach provides a suitable framework for the design of classification systems and that automating the mass function design and calculation would increase the viability of the algorithm for complex classification problems. |
In this paper, the Dempster–Shafer (D–S) method is used as the theoretical basis for | |
Keywords | Dempster–Shafer theory; Data classification |
Year | 2014 |
Journal | Journal of Experimental & Theoretical Artificial Intelligence |
Publisher | Taylor & Francis |
ISSN | 0952813X |
13623079 | |
Digital Object Identifier (DOI) | https://doi.org/10.1080/0952813X.2014.886301 |
Web address (URL) | http://hdl.handle.net/10545/622385 |
hdl:10545/622385 | |
Publication dates | 26 Feb 2014 |
Publication process dates | |
Deposited | 19 Mar 2018, 16:20 |
Rights | Archived with thanks to Journal of Experimental & Theoretical Artificial Intelligence |
Contributors | University of Nottingham |
File | File Access Level Open |
https://repository.derby.ac.uk/item/94578/data-classification-using-the-dempster-shafer-method
Download files
68
total views0
total downloads0
views this month0
downloads this month
Export as
Related outputs
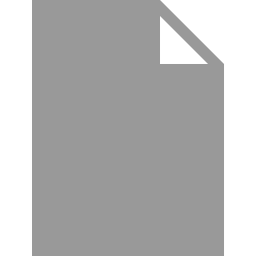
Addressing robustness in time-critical, distributed, task allocation algorithms.
Whitbrook, Amanda, Meng, Qinggang and Chung, Paul W. H. 2018. Addressing robustness in time-critical, distributed, task allocation algorithms. Applied Intelligence. https://doi.org/10.1007/s10489-018-1169-3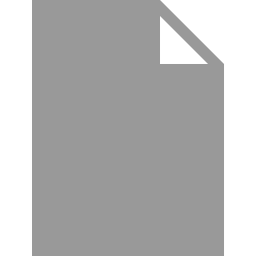
A novel distributed scheduling algorithm for time-critical multi-agent systems.
Whitbrook, Amanda, Meng, Qinggang and Chung, Paul W. H. 2015. A novel distributed scheduling algorithm for time-critical multi-agent systems. IEEE. https://doi.org/10.1109/IROS.2015.7354299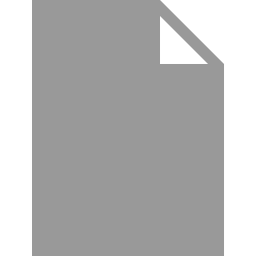
Juxtaposition of system dynamics and agent-based simulation for a case study in immunosenescence.
Figueredo, Grazziela P., Siebers, Peer-Olaf, Aickelin, Uwe, Whitbrook, Amanda and Garibaldi, Jonathan M. 2015. Juxtaposition of system dynamics and agent-based simulation for a case study in immunosenescence. PLos ONE. https://doi.org/10.1371/journal.pone.0118359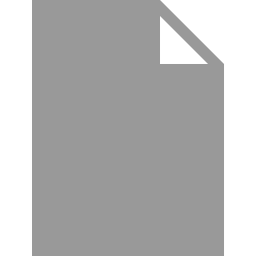
A conceptual framework for combining artificial neural networks with computational aeroacoustics for design development.
McKee, Claire, Harmanto, Dani and Whitbrook, Amanda 2018. A conceptual framework for combining artificial neural networks with computational aeroacoustics for design development. Industrial Engineering and Operations Management Society (IEOM).A cloud-based path-finding framework: Improving the performance of real-time navigation in games
Rowe, Jordan, Whitbrook, Amanda and Chen, Minsi 2017. A cloud-based path-finding framework: Improving the performance of real-time navigation in games. Association of Computing Machinery. https://doi.org/10.1145/3147234.3148097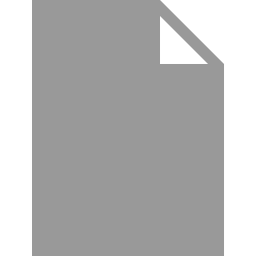
Model building
Lowdnes, Val, Berry, Stuart, Trovati, Marcello and Whitbrook, Amanda 2017. Model building. in: Springer.Distributed task rescheduling with time constraints for the optimization of total task allocations in a multirobot system
Turner, Joanna, Meng, Qinggang, Schaefer, Gerald, Whitbrook, Amanda and Soltoggio, Andrea 2017. Distributed task rescheduling with time constraints for the optimization of total task allocations in a multirobot system. IEEE Transactions on Cybernetics. https://doi.org/10.1109/TCYB.2017.2743164A robust, distributed task allocation algorithm for time-critical, multi agent systems operating in uncertain environments
Whitbrook, Amanda, Meng, Qinggang and Chung, Paul W. H. 2017. A robust, distributed task allocation algorithm for time-critical, multi agent systems operating in uncertain environments.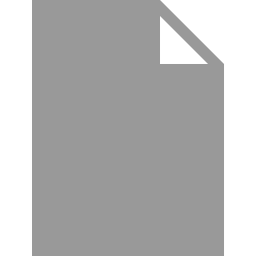