Bringing the Blessing of Dimensionality to the Edge
Conference item
Tyukin, Ivan Y., Gorban, Alexander N, McEwan, Alistair and Meshkinfamfard, Sepehr 2019. Bringing the Blessing of Dimensionality to the Edge. IEEE. https://doi.org/10.1109/iciai.2019.8850825
Authors | Tyukin, Ivan Y., Gorban, Alexander N, McEwan, Alistair and Meshkinfamfard, Sepehr |
---|---|
Abstract | In this work we present a novel approach and algorithms for equipping Artificial Intelligence systems with capabilities to become better over time. A distinctive feature of the approach is that, in the supervised setting, the approaches' computational complexity is sub-linear in the number of training samples. This makes it particularly attractive in applications in which the computational power and memory are limited. The approach is based on the concentration of measure effects and stochastic separation theorems. The algorithms are illustrated with examples. |
Keywords | stochastic separation theorems; supervised learning |
Year | 2019 |
Journal | 2019 1st International Conference on Industrial Artificial Intelligence (IAI) |
International Conference on Industrial Artificial Intelligence (IAI) | |
Publisher | IEEE |
Digital Object Identifier (DOI) | https://doi.org/10.1109/iciai.2019.8850825 |
Web address (URL) | http://hdl.handle.net/10545/625662 |
http://creativecommons.org/publicdomain/zero/1.0/ | |
hdl:10545/625662 | |
ISBN | 9781728135939 |
File | File Access Level Open |
File | File Access Level Open |
File | File Access Level Open |
Publication dates | 30 Sep 2019 |
Publication process dates | |
Deposited | 22 Mar 2021, 14:17 |
Accepted | 01 Jul 2019 |
Rights | CC0 1.0 Universal |
Contributors | University of Leicester and Lobachevsky University, Russia |
Permalink -
https://repository.derby.ac.uk/item/9426y/bringing-the-blessing-of-dimensionality-to-the-edge
Download files
File
![]() | license.txt | |
File access level: Open |
![]() | license_rdf | |
File access level: Open |
08850825.pdf | ||
File access level: Open |
61
total views26
total downloads7
views this month0
downloads this month
Export as
Related outputs
A robust feature adaptation approach against variation of muscle contraction forces for myoelectric pattern recognition-based gesture characterization
Samuel, O., Asogbon, M. and McEwan, A. 2024. A robust feature adaptation approach against variation of muscle contraction forces for myoelectric pattern recognition-based gesture characterization. Biomedical Signal Processing and Control. 95 (2024), p. 106446. https://doi.org/https://doi.org/10.1016/j.bspc.2024.106446A Multi-Dataset Characterization of Window-based Hyperparameters for Deep CNN-driven sEMG Pattern Recognition
Kulwa, F., Zhang, H., Samuel, O., Asogbon, M., Scheme, E., Kushaba, R., McEwan, A. and Li, G. 2023. A Multi-Dataset Characterization of Window-based Hyperparameters for Deep CNN-driven sEMG Pattern Recognition. IEEE Transactions on Human-Machine Systems. pp. 1-12. https://doi.org/10.1109/THMS.2023.3329536An Attention-based Bidirectional LSTM Model for Continuous Cross-subject Estimation of Knee Joint Angle during Running from sEMG Signals
Zangene, A., Samuel, O., Abbasi, A., Nazarpour, K., McEwan, A. and Li, G. 2023. An Attention-based Bidirectional LSTM Model for Continuous Cross-subject Estimation of Knee Joint Angle during Running from sEMG Signals. 45th Annual International Conference of the IEEE, Engineering in Medicine and Biology Society. IEEE. https://doi.org/10.1109/EMBC40787.2023.10340791Enhanced Deep Transfer Learning Model based on Spatial-Temporal driven Scalograms for Precise Decoding of Motor Intent in Stroke Survivors
Samuel, O., Asogbon, M., Kulwa, F., Zangene, A., Oyemakinde, T., Igbe, T., McEwan, A., Li, Y. and Li, G. 2023. Enhanced Deep Transfer Learning Model based on Spatial-Temporal driven Scalograms for Precise Decoding of Motor Intent in Stroke Survivors. 45th Annual International Conference of the IEEE Engineering in Medicine and Biology Society. IEEE. https://doi.org/10.1109/EMBC40787.2023.10340683An efficient attention-driven deep neural network approach for continuous estimation of knee joint kinematics via sEMG signals during running
Zangene, A. R., Samuel, O., Abbasi, A., McEwan, A., Asogbon, M. G., Li, G. and Nazarpour, K. 2023. An efficient attention-driven deep neural network approach for continuous estimation of knee joint kinematics via sEMG signals during running. Biomedical Signal Processing and Control. 86 (B), pp. 1-12. https://doi.org/10.1016/j.bspc.2023.105103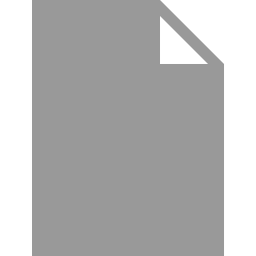