Ensemble of ESA/AATSR aerosol optical depth products based on the likelihood estimate method with uncertainties
Journal article
Authors | Xie, Yanqing, Xue, Yong, Che, Yahui, Guang, Jie, Mei, Linlu, Voorhis, Dave, Fan, Cheng, She, Lu and Xu, Hui |
---|---|
Abstract | Within the European Space Agency Climate Change Initiative (CCI) project Aerosol_cci, there are three aerosol optical depth (AOD) data sets of Advanced Along-Track Scanning Radiometer (AATSR) data. These are obtained using the ATSR-2/ATSR dual-view aerosol retrieval algorithm (ADV) by the Finnish Meteorological Institute, the Oxford-Rutherford Appleton Laboratory (RAL) Retrieval of Aerosol and Cloud (ORAC) algorithm by the University of Oxford/RAL, and the Swansea algorithm (SU) by the University of Swansea. The three AOD data sets vary widely. Each has unique characteristics: the spatial coverage of ORAC is greater, but the accuracy of ADV and SU is higher, so none is significantly better than the others, and each has shortcomings that limit the scope of its application. To address this, we propose a method for converging these three products to create a single data set with higher spatial coverage and better accuracy. The fusion algorithm consists of three parts: the first part is to remove the systematic errors; the second part is to calculate the uncertainty and fusion of data sets using the maximum likelihood estimate method; and the third part is to mask outliers with a threshold of 0.12. The ensemble AOD results show that the spatial coverage of fused data set after mask is 148%, 13%, and 181% higher than those of ADV, ORAC, and SU, respectively, and the root-mean-square error, mean absolute error, mean bias error, and relative mean bias are superior to those of the three original data sets. Thus, the accuracy and spatial coverage of the fused AOD data set masked with a threshold of 0.12 are improved compared to the original data set. Finally, we discuss the selection of mask thresholds. |
Keywords | Remote sensing; Satellites; Aerosol optical depth; Optical surface waves |
Year | 2017 |
Journal | IEEE Transactions on Geoscience and Remote Sensing |
Publisher | IEEE |
ISSN | 01962892 |
15580644 | |
Digital Object Identifier (DOI) | https://doi.org/10.1109/TGRS.2017.2757910 |
Web address (URL) | http://hdl.handle.net/10545/621924 |
http://creativecommons.org/licenses/by/4.0/ | |
hdl:10545/621924 | |
Publication dates | 20 Oct 2017 |
Publication process dates | |
Deposited | 27 Oct 2017, 15:27 |
Rights | Archived with thanks to IEEE Transactions on Geoscience and Remote Sensing |
Contributors | University of Chinese Academy of Sciences, University of Derby, University of Bremen and Chinese Academy of Meteorological Sciences |
File | File Access Level Open |
File | File Access Level Open |
https://repository.derby.ac.uk/item/92355/ensemble-of-esa-aatsr-aerosol-optical-depth-products-based-on-the-likelihood-estimate-method-with-uncertainties
Download files
41
total views0
total downloads2
views this month0
downloads this month
Export as
Related outputs
An Improved Geographically and Temporally Weighted Regression for Surface Ozone Estimation from Satellite-Based Precursor Data
Wang, X., Xue, Y., Sun, Y., Jin, C. and Wu, S. 2023. An Improved Geographically and Temporally Weighted Regression for Surface Ozone Estimation from Satellite-Based Precursor Data. IEEE Journal of Selected Topics in Applied Earth Observations and Remote Sensing. pp. 1-14. https://doi.org/10.1109/JSTARS.2023.3327881Studying the Regional Transmission of Air Pollution Based on Spatiotemporal Multivariable Data
Lu, X., Xue, Y., He, B., Jiang, X., Wu, S. and Wang, X. 2023. Studying the Regional Transmission of Air Pollution Based on Spatiotemporal Multivariable Data. Atmosphere. 14 (9), pp. 1-17. https://doi.org/10.3390/atmos14091438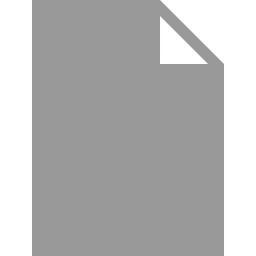
Improved Bi-Angle Aerosol Optical Depth Retrieval Algorithm from AHI Data Based on Particle Swarm Optimization
Jin, Chunlin, Jiang, Xingxing, Sun, Yuxin, Wu, Shuhui and Xue, Yong 2021. Improved Bi-Angle Aerosol Optical Depth Retrieval Algorithm from AHI Data Based on Particle Swarm Optimization. Remote Sensing. 13 (22), p. 4689. https://doi.org/10.3390/rs13224689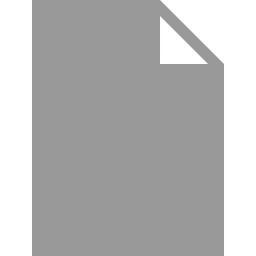
Estimation of the PM2.5 and PM10 Mass Concentration over Land from FY-4A Aerosol Optical Depth Data
Xue, Yong 2021. Estimation of the PM2.5 and PM10 Mass Concentration over Land from FY-4A Aerosol Optical Depth Data. Remote Sensing. 13 (21), p. 4276. https://doi.org/10.3390/rs13214276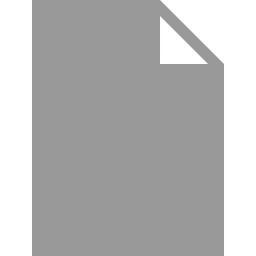
COVID-19 pandemic decision support system for a population defense strategy and vaccination effectiveness
Varotsos, Costas A, Krapivin, Vladimir F, Xue, Yong, Soldatov, Vladimir and Voronova, Tatiana 2021. COVID-19 pandemic decision support system for a population defense strategy and vaccination effectiveness. Safety Science. 142, p. 105370. https://doi.org/10.1016/j.ssci.2021.105370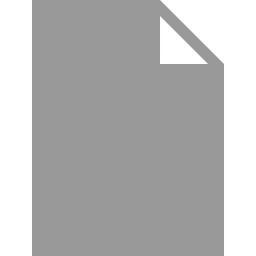
Nowcasting of air pollution episodes in megacities: A case study for Athens, Greece
Varotsos, Costas A., Mazei, Yuri, Saldaev, Damir, Efstathiou, Maria, Voronova, Tatiana and Xue, Yong 2021. Nowcasting of air pollution episodes in megacities: A case study for Athens, Greece. Atmospheric Pollution Research. 12 (7), p. 101099. https://doi.org/10.1016/j.apr.2021.101099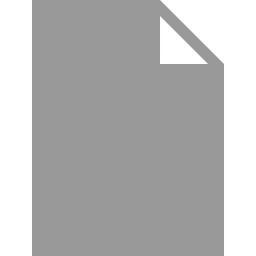
Remote sensing evaluation of total suspended solids dynamic with markov model: a case study of inland reservoir across administrative boundary in south China
Zhao, Jing, Zhang, Fujie, Chen, Shuisen, Wang, Chongyang, Chen, Jinyue, Zhou, Hui and Xue, Yong 2020. Remote sensing evaluation of total suspended solids dynamic with markov model: a case study of inland reservoir across administrative boundary in south China. Sensors. 20 (23), p. 6911. https://doi.org/10.3390/s20236911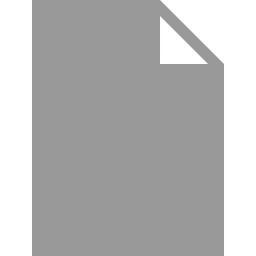
Diagnostic model for the society safety under COVID-19 pandemic conditions
Varotsos, Costas A., Krapivin, Vladimir F. and Xue, Yong 2021. Diagnostic model for the society safety under COVID-19 pandemic conditions. Safety Science. 136, p. 105164. https://doi.org/10.1016/j.ssci.2021.105164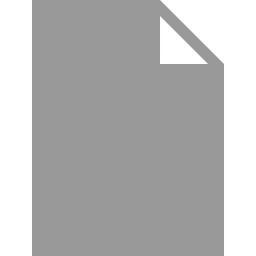
Polarimetric SAR image semantic segmentation with 3D discrete wavelet transform and Markov random field
Bi, Haixia, Xu, Lin, Cao, Xiangyong, Xue, Yong and Xu, Zongben 2020. Polarimetric SAR image semantic segmentation with 3D discrete wavelet transform and Markov random field. IEEE Transactions on Image Processing. https://doi.org/10.1109/TIP.2020.2992177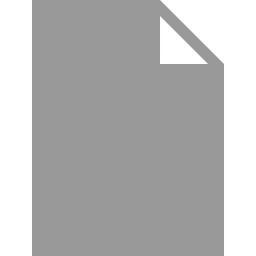
Deriving a global and hourly data set of aerosol optical depth over land using data from four geostationary satellites: goes-16, msg-1, msg-4, and himawari-8
Xie, Yanqing, Xue, Yong, Guang, Jie, Mei, Linlu, She, Lu, Li, Ying, Che, Yahui and Fan, Cheng 2019. Deriving a global and hourly data set of aerosol optical depth over land using data from four geostationary satellites: goes-16, msg-1, msg-4, and himawari-8. IEEE Transactions on Geoscience and Remote Sensing. https://doi.org/10.1109/TGRS.2019.2944949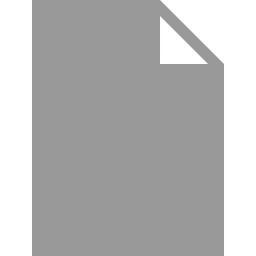
An active deep learning approach for minimally supervised polsar image classification
Xue, Yong 2019. An active deep learning approach for minimally supervised polsar image classification. IEEE Transactions on Geoscience and Remote Sensing. 57 (11), pp. 9378-9395. https://doi.org/10.1109/TGRS.2019.2926434A Heterogeneous and Interactive Big Earth Data Framework
Bi, H., Xue, Y., Merritt, P., Windmill, C. and Davis, B. 2019. A Heterogeneous and Interactive Big Earth Data Framework. 2019 International Conference on Big Data Engineering. IEEE. https://doi.org/10.1145/3341620.3341628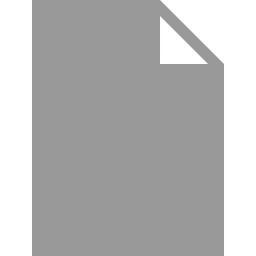
Big earth data: a comprehensive analysis of visualization analytics issues
Merritt, Patrick, Bi, Haixia, Davis, Bradley, Windmill, Christopher and Xue, Yong 2019. Big earth data: a comprehensive analysis of visualization analytics issues. Big Earth Data. 2 (4), pp. 321-350. https://doi.org/10.1080/20964471.2019.1576260High Performance Time Series Quantitative Retrieval from Satellite Images on a GPU Cluster
Liu, J., Xue, Y., Ren, K., Song, J., Windmill, C. and Merritt, P. 2019. High Performance Time Series Quantitative Retrieval from Satellite Images on a GPU Cluster. IEEE Journal of Selected Topics in Applied Earth Observations and Remote Sensing. 12 (8). https://doi.org/10.1109/JSTARS.2019.2920077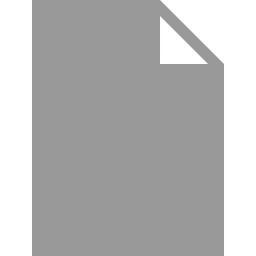
Joint retrieval of aerosol optical depth and surface reflectance over land using geostationary satellite data.
She, Lu, Xue, Yong, Yang, Xihua, Leys, John, Guang, Jie, Che, Yahui, Fan, Cheng, Xie, Yanqing and Li, Ying 2018. Joint retrieval of aerosol optical depth and surface reflectance over land using geostationary satellite data. IEEE Transactions on Geoscience and Remote Sensing. https://doi.org/10.1109/TGRS.2018.2867000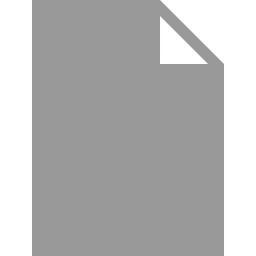
Evaluation of the AVHRR DeepBlue aerosol optical depth dataset over mainland China.
Che, Yahui, Xue, Yong, Guang, Jie, She, Lu and Guo, Jianping 2018. Evaluation of the AVHRR DeepBlue aerosol optical depth dataset over mainland China. ISPRS Journal of Photogrammetry and Remote Sensing. https://doi.org/10.1016/j.isprsjprs.2018.09.004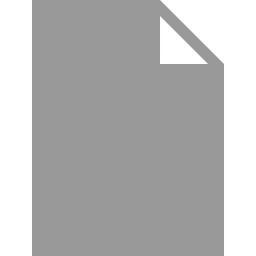
A physically based PM 2.5 estimation method using AERONET data in Beijing Area
Chen, Guili, Guang, Jie, Xue, Yong, Li, Ying, Che, Yahui and Gong, Shaoqi 2018. A physically based PM 2.5 estimation method using AERONET data in Beijing Area. IEEE Journal of Selected Topics in Applied Earth Observations and Remote Sensing. https://doi.org/10.1109/JSTARS.2018.2817243Dust detection and intensity estimation using Himawari-8/AHI observation.
She, Lu, Xue, Yong, Yang, Xihua, Guang, Jie, Li, Ying, Che, Yahui, Fan, Cheng and Xie, Yanqing 2018. Dust detection and intensity estimation using Himawari-8/AHI observation. Remote Sensing. https://doi.org/10.3390/rs10040490SAHARA: A Simplified AtmospHeric Correction AlgoRithm for Chinese gAofen Data: 1. Aerosol Algorithm.
She, Lu, Mei, Linlu, Xue, Yong, Che, Yahui and Guang, Jie 2017. SAHARA: A Simplified AtmospHeric Correction AlgoRithm for Chinese gAofen Data: 1. Aerosol Algorithm. Remote Sensing. https://doi.org/10.3390/rs9030253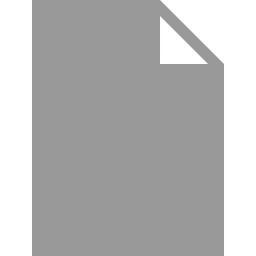
Validation of aerosol products from AATSR and MERIS/AATSR synergy algorithms—Part 1: Global Evaluation.
Che, Yahui, Mei, Linlu, Xue, Yong, Guang, Jie, She, Lu and Li, Ying 2018. Validation of aerosol products from AATSR and MERIS/AATSR synergy algorithms—Part 1: Global Evaluation. Remote Sensing. https://doi.org/10.3390/rs10091414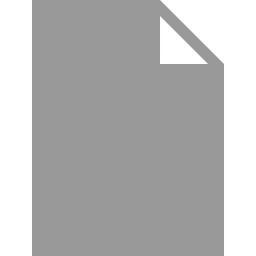
Rel: The 'true' relational model for desktop data processing.
Voorhis, Dave 2017. Rel: The 'true' relational model for desktop data processing. University of Derby.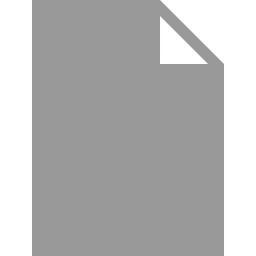
Tools and technologies for the implementation of Big Data.
Self, Richard and Voorhis, Dave 2015. Tools and technologies for the implementation of Big Data. in: Elsevier.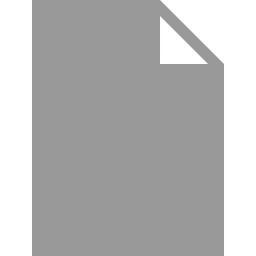
Improved aerosol optical depth and ångstrom exponent retrieval over land From MODIS based on the non-lambertian forward model
Leiku, Yang, Xue, Yong, Guang, Jie, Hassan, Kazemian, Zhang, Jiahua and Li, Chi 2014. Improved aerosol optical depth and ångstrom exponent retrieval over land From MODIS based on the non-lambertian forward model. IEEE Geoscience and Remote Sensing Letters. https://doi.org/10.1109/LGRS.2014.2303317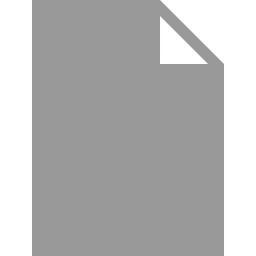
Using SeaWiFS measurements to evaluate radiometric stability of pseudo-invariant calibration sites at top of atmosphere
Li, Chi, Xue, Yong, Liu, Quanhua, Ouazzane, Karim and Zhang, Jiahua 2014. Using SeaWiFS measurements to evaluate radiometric stability of pseudo-invariant calibration sites at top of atmosphere. IEEE Geoscience and Remote Sensing Letters. https://doi.org/10.1109/LGRS.2014.2329138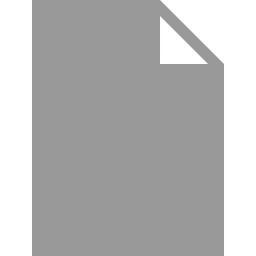
Long-time series aerosol optical depth retrieval from AVHRR data over land in North China and Central Europe
Xue, Yong, He, Xingwei, de Leeuw, Gerrit, Mei, Linlu, Che, Yahui, Rippin, Wayne, Guang, Jie and Hu, Yincui 2017. Long-time series aerosol optical depth retrieval from AVHRR data over land in North China and Central Europe. Remote Sensing of Environment. https://doi.org/10.1016/j.rse.2017.06.036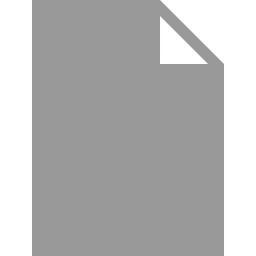
Multicore processors and graphics processing unit accelerators for parallel retrieval of aerosol optical depth from satellite data: Implementation, performance, and energy efficiency
Liu, Jia, Feld, Dustin, Xue, Yong, Garcke, Jochen and Soddemann, Thomas 2015. Multicore processors and graphics processing unit accelerators for parallel retrieval of aerosol optical depth from satellite data: Implementation, performance, and energy efficiency. IEEE Journal of Selected Topics in Applied Earth Observations and Remote Sensing. https://doi.org/10.1109/JSTARS.2015.2438893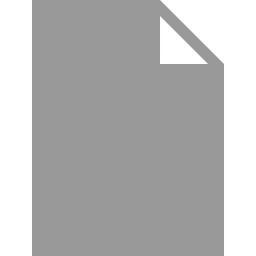
High-throughput geocomputational workflows in a grid environment
Liu, Jia, Xue, Yong, Palmer-Brown, Dominic, Chen, Ziqiang and He, Xingwei 2015. High-throughput geocomputational workflows in a grid environment. Computer. https://doi.org/10.1109/MC.2015.331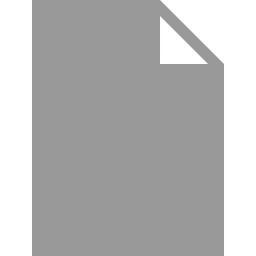
An efficient geosciences workflow on multi-core processors and GPUs: a case study for aerosol optical depth retrieval from MODIS satellite data
Liu, Jia, Feld, Dustin, Xue, Yong, Garcke, Jochen, Soddemann, Thomas, Pan, Peiyuan and Fraunhofer Institute of Algorhithms and Scientific Computing 2016. An efficient geosciences workflow on multi-core processors and GPUs: a case study for aerosol optical depth retrieval from MODIS satellite data. International Journal of Digital Earth. https://doi.org/10.1080/17538947.2015.1130087Technical note: Intercomparison of three AATSR Level 2 (L2) AOD products over China
Che, Yahui, Xue, Yong, Mei, Linlu, Guang, Jie, She, Lu, Guo, Jianping, Hu, Yincui, Xu, Hui, He, Xingwei, Di, Aojie and Fan, Cheng 2016. Technical note: Intercomparison of three AATSR Level 2 (L2) AOD products over China. Atmospheric Chemistry and Physics. https://doi.org/10.5194/acp-16-9655-2016Dust aerosol optical depth retrieval and dust storm detection for Xinjiang Region using Indian National Satellite Observations
Di, Aojie, Xue, Yong, Yang, Xihua, Leys, John, Guang, Jie, Mei, Linlu, Wang, Jingli, She, Lu, Hu, Yincui, He, Xingwei, Che, Yahui and Fan, Cheng 2016. Dust aerosol optical depth retrieval and dust storm detection for Xinjiang Region using Indian National Satellite Observations. Remote Sensing. https://doi.org/10.3390/rs8090702Grid workflow validation using ontology-based tacit knowledge: A case study for quantitative remote sensing applications
Liu, Jia, Liu, Longli, Xue, Yong, Dong, Jing, Hu, Yincui, Hill, Richard, Guang, Jie and Li, Chi 2016. Grid workflow validation using ontology-based tacit knowledge: A case study for quantitative remote sensing applications. Computers & Geosciences. https://doi.org/10.1016/j.cageo.2016.10.002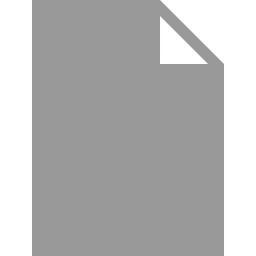
Rel
Voorhis, Dave 2016. Rel.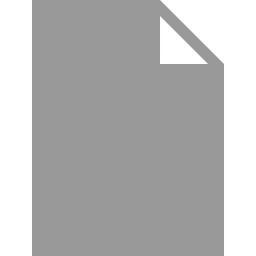
From DOOM to duty: The evolution of design patterns in first person shooters
Voorhis, Dave and Thompson, Tommy 2016. From DOOM to duty: The evolution of design patterns in first person shooters. Digital Games Research Association.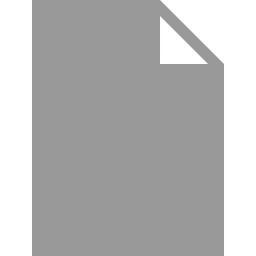
Tools and technologies for the implementation of Big Data
Self, Richard and Voorhis, Dave 2015. Tools and technologies for the implementation of Big Data. in: Butterworth-Heinemann.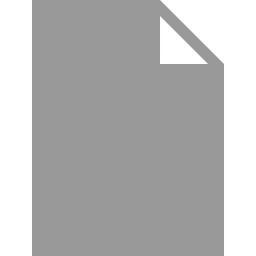
Rel
Voorhis, Dave 2004. Rel.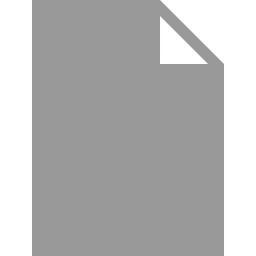
Planning in the Cloud: Massively Parallel Planning
Voorhis, Dave and Thompson, Tommy 2014. Planning in the Cloud: Massively Parallel Planning. IEEE Computer Society. https://doi.org/10.1109/UCC.2014.67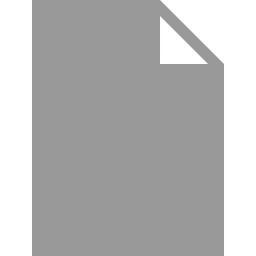
Designing Big Data Analytics Undergraduate and Postgraduate Programmes for Employability
Self, Richard and Voorhis, Dave 2014. Designing Big Data Analytics Undergraduate and Postgraduate Programmes for Employability.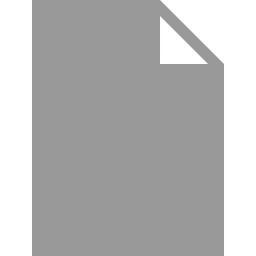
Novel Approaches to Learning and Teaching SAS-based Analytics
Self, Richard and Voorhis, Dave 2013. Novel Approaches to Learning and Teaching SAS-based Analytics. BDA EdCon.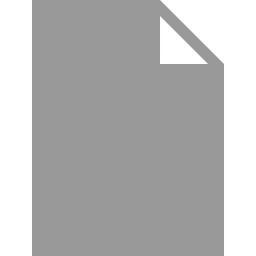